TBCOV: Two Billion Multilingual COVID-19 Tweets with Sentiment, Entity, Geo, and Gender Labels |
This respository provides access to the TBCOV dataset comprising more than two billion multilingual tweets related to the COVID-19 pandemic. Specifically, TBCOV offers 2,014,792,896 tweets collected using more than 800 multilingual keywords over a 14-month period from February 1st, 2020 till March 31st, 2021. These tweets span 67 international languages, posted by 87 million unique users across 218 countries worldwide. Please note that the data shared on this repository is for the sole purpose of non-commercial research.
Paper:
Please cite the following paper, if you use this dataset in your derived research: Muhammad Imran, Umair Qazi, Ferda Ofli. TBCOV: Two Billion Multilingual COVID-19 Tweets with Sentiment, Entity, Geo, and Gender Labels. Data. 2022; 7(1):8. https://doi.org/10.3390/data7010008. [arXiv | bibtex]Worldwide tweets normalized by total population in each county (per 100,000 persons)
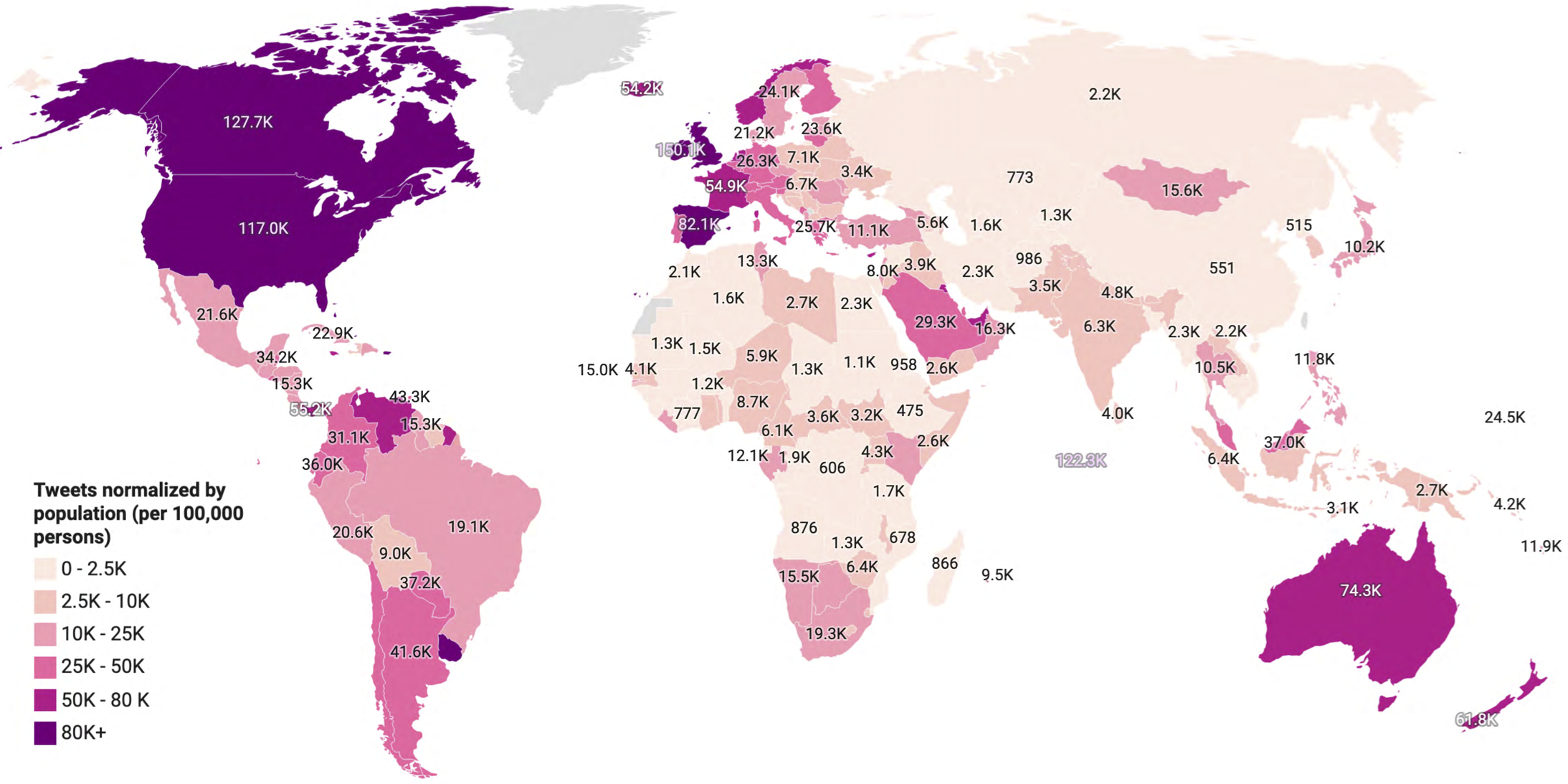
Weekly distribution of two billion tweets (1-Feb-2020 to 31-March-2021)
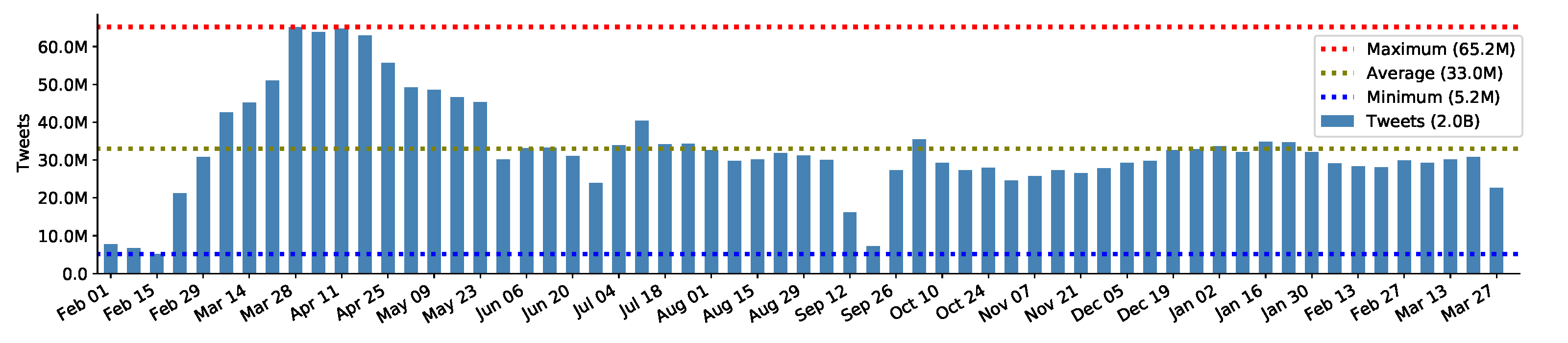
General statistics:
Type | Number |
---|---|
Total tweets | 2,014,792,896 |
Unique users | 87,771,834 |
Countries covered | 218 |
Cities covered | 24,424 |
Languages covered | 67 |
Geographical information statistics:
Type | Number |
---|---|
Tweets with geo-coordinates | 2,799,378 |
Tweets with place information | 51,411,442 |
Tweets with user location information | 1,284,668,011 |
Tweets with location mentions in user profile description | 180,508,901 |
Tweets with location mentions in tweet-text | 600,185,738 |
Keywords and hashtags used for data collection
More than 800 hashtags and keywords were used for the data collection. Below we list some of them. The complete set of keywords can be found here: keywords
Covid_19, #COVID_19uk, Covid19_DE, #covid19Canada, Covid19DE, Covid19Deutschland, #covid19espana, #covid19france, #covid19Indonesia, #covid19ireland, #covid19uk, #ForcaCoronaVirus, #infocoronavirus, #kamitidaktakutviruscorona, #nCoV, nCoV, #ncov2019, nCoV2019, NeuerCoronavirus, #NeuerCoronavirus, Nouveau coronavirus, #NouveauCoronavirus, novel coronavirus, #SARSCoV2, #SARSCoV2, فيروس كورونا, #فيروس_كورونا, #كورونا, #كورونا_الجديد, #कोरोना, कोरोना, कोरोना वायरस, #कोरोना_वायरस, 코로나, #코로나, #코로나19, 코로나바이러스, #코로나바이러스, コロナ, #コロナ, #コロナウイルス, 加油武汉, #加油武汉, #新冠病毒, 新冠病毒, #新冠肺炎, 新冠肺炎, #新型コロナウイルス, #新型冠状病毒, 新型冠状病毒, 武汉加油, #武汉加油, #武汉疫情, #武汉肺炎, 武汉肺炎, #武漢肺炎, 武漢肺炎, 疫情, #疫情, #CoronaAlert, #coronavirusUP, #coronavirustelangana, #coronaviruskerala, #coronavirusmumbai, #coronavirusdelhi, #coronavirusmaharashtra, #coronavirusinindia, वूहान, #कोविड_19, #कोविड-१९, #कोरोनावायरस, breathing issues, breathing difficulties, fever, cough, pandemic, Coronavirus usa, Coronavirus US, #coronaviruscalifornia, #CoronaVirusCA, #CoronaVirusSeattle, #Coronavirusnyc , #coronavirusnewyork, #Coronavirustexas, Coronavirus, #Coronavirus, #CoronavirusOutbreak, #2019nCov, coronavirus outbreak, COVID-19, #CoronaVirusitaly, CoronaVirus Iran, CoronaVirus Korean, CoronaVirus Japan, Corona, Coronavirus, Corona virus كورونا, #كورونا, #فيروس_كورونا, #كورونا_الجديد, #فيروس_كورونا_المستجد, #كورونا_مصر, #كورونا_قطر, #كورونا_السعودية, #كورونا_العراق, #كورونا_إيران, #كورونا_البحرين, #كورونا_الأردن, #كورونا_لبنان, #كورونا_الكويت
#socialdistancing, social distancing, #NovelCorona, novelcoronavirus, #ohiocoronavirus, #PánicoPorCoranovirus, social distance, coronavirus, #N95, #Swiss, #coronavirus, Italia, lombardia, #covid19italia, covid19, corona virus, coronavirus, #covid-19, #corona, #coronavirus
Afghanistan Coronavirus, Albania Coronavirus, Algeria Coronavirus, Andorra Coronavirus, Angola Coronavirus, Argentina Coronavirus, Armenia Coronavirus, Australia Coronavirus, Austria Coronavirus, Azerbaijan Coronavirus, Bahamas Coronavirus, Bahrain Coronavirus, Bangladesh Coronavirus, Barbados Coronavirus, Belarus Coronavirus, Belgium Coronavirus, Belize Coronavirus, Benin Coronavirus, Bhutan Coronavirus, Bolivia Coronavirus, Bosnia Herzegovina Coronavirus, Botswana Coronavirus, Brazil Coronavirus, Brunei Coronavirus, Bulgaria Coronavirus, Burkina Coronavirus, Burundi Coronavirus, Cambodia Coronavirus, Cameroon Coronavirus, Canada Coronavirus, Cape Verde Coronavirus, Central African Rep Coronavirus, Chad Coronavirus, Chile Coronavirus, China Coronavirus, Colombia Coronavirus, Comoros Coronavirus, Congo Coronavirus, Congo Coronavirus, Costa Rica Coronavirus, Croatia Coronavirus, Cuba Coronavirus, Cyprus Coronavirus, Czech Republic Coronavirus, Denmark Coronavirus, Djibouti Coronavirus, Dominica Coronavirus, Dominican Republic Coronavirus, East Timor Coronavirus, Ecuador Coronavirus, Egypt Coronavirus, El Salvador Coronavirus, Equatorial Guinea Coronavirus, Eritrea Coronavirus, Estonia Coronavirus, Ethiopia Coronavirus, Fiji Coronavirus, Finland Coronavirus, France Coronavirus,....
Qatar COVID-19, Romania COVID-19, Russian Federation COVID-19, Rwanda COVID-19, St Lucia COVID-19, Samoa COVID-19, San Marino COVID-19, Saudi Arabia COVID-19, Senegal COVID-19, Serbia COVID-19, Seychelles COVID-19, Sierra Leone COVID-19, Singapore COVID-19, Slovakia COVID-19, Slovenia COVID-19, Solomon Islands COVID-19, Somalia COVID-19, South Africa COVID-19, South Sudan COVID-19, Spain COVID-19, Sri Lanka COVID-19, Sudan COVID-19, Suriname COVID-19, Swaziland COVID-19, Sweden COVID-19, Switzerland COVID-19, Syria COVID-19, Taiwan COVID-19, Tajikistan COVID-19, Tanzania COVID-19, Thailand COVID-19, Togo COVID-19, Tonga COVID-19, Tunisia COVID-19, Turkey COVID-19, Turkmenistan COVID-19, Tuvalu COVID-19, Uganda COVID-19, Ukraine COVID-19, United Arab Emirates COVID-19, UAE COVID-19, United Kingdom COVID-19, UK COVID-19, United States COVID-19, Uruguay COVID-19, Uzbekistan COVID-19, Vanuatu COVID-19, Vatican City COVID-19, Venezuela COVID-19, Vietnam COVID-19, Yemen COVID-19, Zambia COVID-19, Zimbabwe COVID-19, ....
Download
We offer different types of data releases, which provide access to an extensive set of attributes (N=37) ranging from tweet-ids and user-ids to sentiment labels, named-entities, geotags, and gender information in tab-separated values (TSV) files. All the attributes and their datatypes are described in the data descriptor section below.
Monthly release:
The monthly data release provides access to all two billion tweets. It consists of 14 compressed (TAR.GZ) files representing the 14-months data collection period starting Feb-2020 till March-2021. Each zip file may contain several TSV files where a TSV file contains a maximum of 20 million tweets.
DownloadCountry release:
The country data release provides access to country-specific tweets. TBCOV dataset contains tweets from 218 countries. The following dropdown provide access to individual countries data.
DownloadLanguage release:
The language data release provides access to language-specific tweets. TBCOV contains tweets from 67 languages. The dropdown below provide access to individual language-specific files. Moreover, we provide tweets with "Unknown" language, hopeing it's useful for language-detection tasks.
DownloadSpecial data requests
If the above-mentioned data releases do not fulfil your needs, you can send us your request here: REQUEST DATASETData descriptor
The TSV files in all data releases contain the following attributes:Attribute | Type | Description |
---|---|---|
tweet_id |
Int64 | The integer representation of the unique identifier of a tweet. This number is greater than 53 bits and some programming languages may have difficulty/silent defects in interpreting it. |
date_time |
String | UTC time when the tweet was created. |
lang |
String | ISO-6391 Alpha-2 language code consisting of two characters. |
user_id |
String | Represents the id of the author of the tweet. |
retweeted_id |
Int64 | If the tweet is a retweet, the retweeted_id represents the id of the parent tweet. |
quoted_id |
Int64 | If the tweet is a quoted tweet, the quoted_id represents the id of the parent tweet. |
in_reply_to_id |
Int64 | If the tweet is a reply to an existing tweet, the in_reply_to_id represents the id of the parent/original tweet. |
sentiment_label |
Int64 | Represents the sentiment label values: -1 (negative), 0 (neutral), 1 (positive). |
sentiment_conf |
Float | Represents the confidence score of the sentiment classifier for a given sentiment label to a tweet. |
user_type |
String | Represents the inferred type of the user account. Personal accounts as coded as "PER" and accounts belonging to organizations are coded as "ORG". |
gender_label |
String | One character code representing the identified gender of the user where "F" represents female and "M" represents male user types. |
tweet_text_named_entities |
Dictionary array | Named-entities (person, organization, location, etc.) extracted from tweet text are provided in this attribute in the array of dictionary format. The details of all named entities can be found below in a table. |
geo_coordinates_lat_lon |
Float | GPS coordinates in the latitude, longitude format retrieved from the user's GPS-enabled device. |
geo_country_code |
String | Two characters country code obtained through resolving the GPS coordinates (latitude, longitude). |
geo_state |
String | The name of the state/province obtained through resolving the GPS coordinates (latitude, longitude). |
geo_county |
String | The name of the county obtained through resolving the GPS coordinates (latitude, longitude). |
geo_city |
String | The name of the city obtained through resolving the GPS coordinates (latitude, longitude). |
place_bounding_box |
Float | Twitter provided bounding boxes representing place tags. |
place_country_code |
String | Two characters country code obtained through resolving the place bounding boxes. |
place_state |
String | The name of the state/province obtained through resolving the place bounding boxes. |
place_county |
String | The name of the county obtained through resolving the place bounding boxes. |
place_city |
String | The name of the city obtained through resolving the place bounding boxes. |
user_loc_toponyms |
Dictionary array | Toponyms recognized and extracted from the user location field provided as an array of dictionary. |
user_loc_country_code |
String | Two characters country code obtained through resolving the user location toponyms. |
user_loc_state |
String | The name of the state/province obtained through resolving the user location toponyms. |
user_loc_county |
String | The name of the county obtained through resolving the user location toponyms. |
user_loc_city |
String | The name of the city obtained through resolving the user location toponyms. |
user_profile_description_toponyms |
Dictionary array | Toponyms recognized and extracted from the user profile description field provided as an array of dictionary. |
user_profile_description_country_code |
String | Two characters country code learned through resolving the recognized user profile description toponyms. |
user_profile_description_state |
String | The name of the state/province obtained through resolving the recognized user profile description toponyms. |
user_profile_description_county |
String | The name of the county obtained through resolving the recognized user profile description toponyms. |
user_profile_description_city |
String | The name of the city learned through resolving the recognized user profile description toponyms. |
tweet_text_toponyms |
Dictionary array | Toponyms recognized and extracted from the tweet full_text field in the dictionary array format. |
tweet_text_country_code |
String | Two characters country code obtained through resolving the recognized tweet text toponyms. |
tweet_text_state |
String | The name of the state/province obtained through resolving the recognized tweet text toponyms. |
tweet_text_county |
String | The name of the county learned through resolving the recognized tweet text toponyms. |
tweet_text_city |
String | The name of the city learned through resolving the recognized tweet text toponyms. |
Named Entities
Entity type | Description |
---|---|
PERSON |
Name of a person. E.g., Peter Pan, Steve Jobs |
ORG |
Campanies, agencies, institites names, e.g, MIT, Microsoft, QCRI |
GPE |
Name of countries, cities, states, etc. |
LOC |
Non-GPE locations such as mountain ranges, water bodies, etc. |
FAC |
Represents buildings, airports, highways, etc. |
PRODUCT |
Objects, vehicles, foods, etc. |
NORP |
Nationalities or religious or political groups |
LANGUAGE |
Any named language e.g., English, Arabic |
DATE |
Dates or periods, e.g., July 12, 2003 |
TIME |
Times smaller than a day. E.g., five hours, 2 hours |
QUANTITY |
Measurements, as of weight or distance. E.g., 40 kg, several kilometers |
CARDINAL |
Numerals such as 8, five, ten |
ORDINAL |
First, second, third, etc. |
PERCENT |
Percentages, including % sign |
EVENT |
Named event names e.g., hurricanes, battles, wars |
MONEY |
Monetary values and unit, e.g., ten cents |
LAW |
Named documents made into laws |
WORK_OF_ART |
Titles of books, songs, etc. |
COVID-ENTITY |
COVID-19 related terms such as corona, covid_19, coronavirus |